
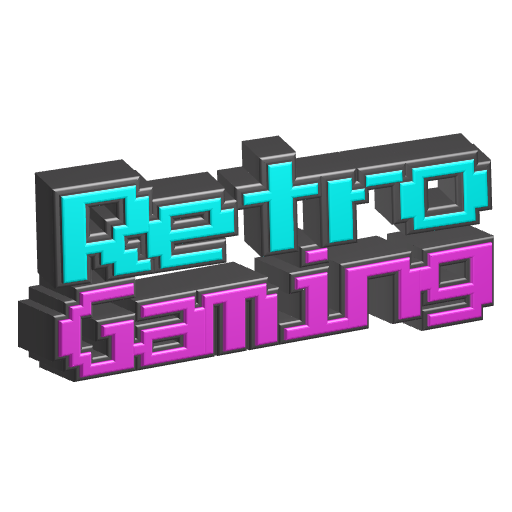
yeah. find the es_input.cfg
file
yeah. find the es_input.cfg
file
On Linux, that’s usually the case. Finding the config file is the problem. I suspect that’s why emulation Station isn’t working. I don’t know where that’s installed, but I’d assume there’s another configuration file for ES. It’s probably in the home directory, ~. maybe ~/.emulation_station or or ~/.ES. I don’t recall, but there will be a file structure similar to the RetroArch tree.
In either case, it would be very kind to post the full solution for the next person.
I’ve never had issues with the 8bitdo Controllers on rpi, Bluetooth or wired, but I found a thread where others solved the same problem. Looks like that particular controller isn’t perfectly supported and you need to update xpad and a configuration file.
I intended B, but A is also true, no?
Yeah. I’m thinking more along the lines of research and open models than anything to do with OpenAI. Fair use, above all else, generally requires that the derivative work not threaten the economic viability of the original and that’s categorically untrue of ChatGPT/Copilot which are marketed and sold as products meant to replace human workers.
The clean room development analogy is definitely an analogy I can get behind, but raises further questions since LLMs are multi stage. Technically, only the tokenization stage will “see” the source code, which is a bit like a “clean room” from the perspective of subsequent stages. When does something stop being just a list of technical requirements and veer into infringement? I’m not sure that line is so clear.
I don’t think the generative copyright thing is so straightforward since the model requires a human agent to generate the input even if the output is deterministic. I know, for example, Microsoft’s Image Generator says that the images fall under creative Commons, which is distinct from public domain given that some rights are withheld. Maybe that won’t hold up in court forever, but Microsoft’s lawyers seem to think it’s a bit more nuanced than “this output can’t be copyrighted”. If it’s not subject to copyright, then what product are they selling? Maybe the court agrees that LLMs and monkeys are the same, but I’m skeptical that that will happen considering how much money these tech companies have poured into it and how much the United States seems to bend over backwards to accommodate tech monopolies and their human rights violations.
Again, I think it’s clear that commerical entities using their market position to eliminate the need for artists and writers is clearly against the spirit of copyright and intellectual property, but I also think there are genuinely interesting questions when it comes to models that are themselves open source or non-commercial.
For example, if I ask it to produce python code for addition, which GPL’d library is it drawing from?
I think it’s clear that the fair use doctrine no longer applies when OpenAI turns it into a commercial code assistant, but then it gets a bit trickier when used for research or education purposes, right?
I’m not trying to be obtuse-- I’m an AI researcher who is highly skeptical of AI. I just think the imperfect compression that neural networks use to “store” data is a bit less clear than copy/pasting code wholesale.
would you agree that somebody reading source code and then reimplenting it (assuming no reverse engineering or proprietary source code) would not violate the GPL?
If so, then the argument that these models infringe on right holders seems to hinge on the verbatim argument that their exact work was used without attribution/license requirements. This surely happens sometimes, but is not, in general, a thing these models are capable of since they’re using loss-y compression to “learn” the model parameters. As an additional point, it would be straightforward to then comply with DMCA requests using any number of published “forced forgetting” methods.
Then, that raises a further question.
If I as an academic researcher wanted to make a model that writes code using GPL’d training data, would I be in compliance if I listed the training data and licensed my resulting model under the GPL?
I work for a university and hate big tech as much as anyone on Lemmy. I am just not entirely sure GPL makes sense here. GPL 3 was written because GPL 2 had loopholes that Microsoft exploited and I suspect their lawyers are pretty informed on the topic.
I hate big tech too, but I’m not really sure how the GPL or MIT licenses (for example) would apply. LLMs don’t really memorize stuff like a database would and there are certain (academic/research) domains that would almost certainly fall under fair use. LLMs aren’t really capable of storing the entire training set, though I admit there are almost certainly edge cases where stuff is taken verbatim.
I’m not advocating for OpenAI by any means, but I’m genuinely skeptical that most copyleft licenses have any stake in this. There’s no static linking or source code distribution happening. Many basic algorithms don’t follow under copyright, and, in practice, stack overflow code is copy/pasted all the time without that being released under any special license.
If your code is on GitHub, it really doesn’t matter what license you provide in the repository – you’ve already agreed to allowing any user to “fork” it for any reason whatsoever.
People who use LLMs to write code (incorrectly) perceived their code to be more secure than code written by expert humans.
and my point was explaining that that work has likely been done because the paper I linked was 20 years old and they talk about the deep connection between “similarity” and “compresses well”. I bet if you read the paper, you’d see exactly why I chose to share it-- particularly the equations that define NID and NCD.
The difference between “seeing how well similar images compress” and figuring out “which of these images are similar” is the quantized, classficiation step which is trivial compared to doing the distance comparison across all samples with all other samples. My point was that this distance measure (using compressors to measure similarity) has been published for at least 20 years and that you should probably google “normalized compression distance” before spending any time implementing stuff, since it’s very much been done before.
I think there’s probably a difference between an intro to computer science course and the PhD level papers that discuss the ability of machines to learn and decide, but my experience in this is limited to my PhD in the topic.
And, no, textbooks are often not peer reviewed in the same way and generally written by graduate students. They have mistakes in them all the time. Or grand statements taken out of context. Or are simplified explanations because introducing the nuances of PAC-learnability to somebody who doesn’t understand a “for” loop is probably not very productive.
I came here to share some interesting material from my PhD research topic and you’re calling me an asshole. It sounds like you did not have a wonderful day and I’m sorry for that.
Did you try learning about how computers learn things and make decisions? It’s pretty neat
You seem very upset, so I hate to inform you that neither one of those are peer reviewed sources and that they are simplifying things.
“Learning” is definitely something a machine can do and then they can use that experience to coordinate actions based on data that is inaccesible to the programmer. If that’s not “making a decision”, then we aren’t speaking the same language. Call it what you want and argue with the entire published field or AI, I guess. That’s certainly an option, but generally I find it useful for words to mean things without getting too pedantic.
Yeah. I understand. But first you have to cluster your images so you know which ones are similar and can then do the deduplication. This would be a powerful way to do that. It’s just expensive compared to other clustering algorithms.
My point in linking the paper is that “the probe” you suggested is a 20 year old metric that is well understood. Using normalized compression distance as a measure of Kolmogorov Complexity is what the linked paper is about. You don’t need to spend time showing similar images will compress more than dissimilar ones. The compression length is itself a measure of similarity.
Yeah. That’s what an MP4 does, but I was just saying that first you have to figure out which images are “close enough” to encode this way.
Then it should be easy to find peer reviewed sources that support that claim.
I found it incredibly easy to find countless articles suggesting that your Boolean is false. Weird hill to die on. Have a good day.
Agree to disagree. Something makes a decision about how to classify the images and it’s certainly not the person writing 10 lines of code. I’d be interested in having a good faith discussion, but repeating a personal opinion isn’t really that. I suspect this is more of a metaphysics argument than anything and I don’t really care to spend more time on it.
I hope you have a wonderful day, even if we disagree.
computers make decisions all the time. For example, how to route my packets from my instance to your instance. Classification functions are well understood in computer science in general, and, while stochastic, can be constructed to be arbitrarily precise.
https://en.wikipedia.org/wiki/Probably_approximately_correct_learning?wprov=sfla1
Human facial detection has been at 99% accuracy since the 90s and OPs task I’d likely a lot easier since we can exploit time and location proximity data and know in advance that 10 pictures taken of Alice or Bob at one single party are probably a lot less variant than 10 pictures taken in different contexts over many years.
What OP is asking to do isn’t at all impossible-- I’m just not sure you’ll save any money on power and GPU time compared to buying another HDD.
Definitely PhD.
It’s very much an ongoing and under explored area of the field.
One of the biggest machine learning conferences is actually hosting a workshop on the relationship between compression and machine learning (because it’s very deep). https://neurips.cc/virtual/2024/workshop/84753
Compressed length is already known to be a powerful metric for classification tasks, but requires polynomial time to do the classification. As much as I hate to admit it, you’re better off using a neural network because they work in linear time, or figuring out how to apply the kernel trick to the metric outlined in this paper.
a formal paper on using compression length as a measure of similarity: https://arxiv.org/pdf/cs/0111054
a blog post on this topic, applied to image classification:
By no means the best option, but the tikz latex package works and pandoc can handle the conversion to your preferred format. I would limit this to very simple diagrams.
You can install Plex on your mobile device and toggle the “share media from this device” setting. Otherwise, a steam deck would have everything an RPI has plus a GPU and a touch screen. Since there are two radios (2 and 5Ghz) on the device, you should be able to set it up as a bridge device, but I’ve not tried this personally.